
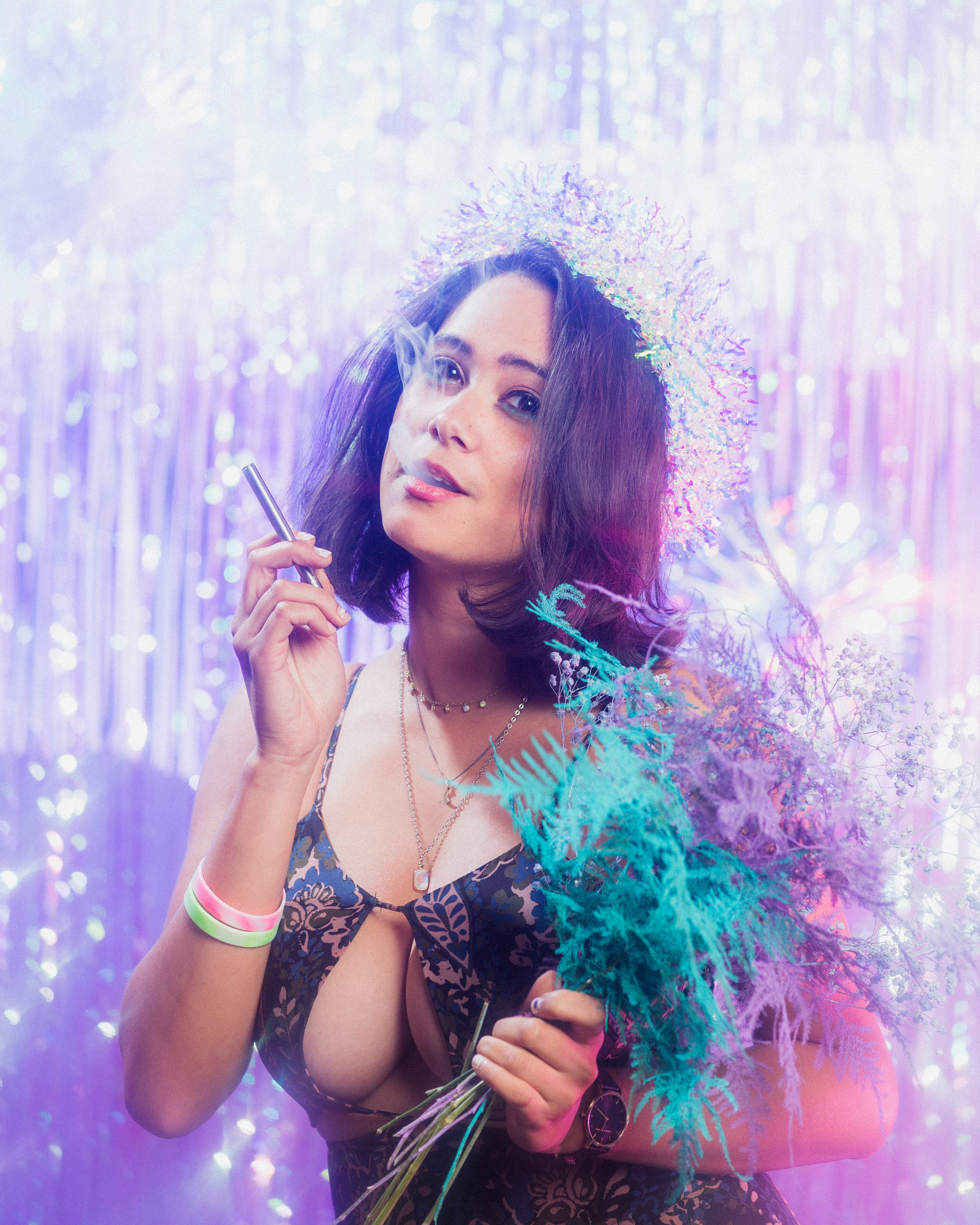
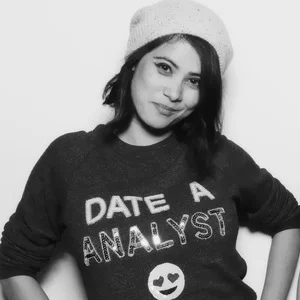
Hi, I’m Jewel. I make music and do product management. And sometimes both at the same time.
I play bass. I do so in too many bands. You can find me taking myself too seriously with Frond. You can find me making puns and dancing like no one is watching with Golden Idols. And you can find me causing a ruckus with The Grizzled Mighty.
Despite what you may have heard, I’m not rolling in cash from the aforementioned musical projects. So, I also work as a product manager, currently at a pretty cool place called Headset, who make tools for cannabis retail data intelligence. Before that I was at Tableau. So, I guess data is kinda my thing.
I like talking about both of these things and have a couple presentations where I talk about them simultaneously.
Without music to decorate it, time is just a bunch of boring production deadlines or dates by which bills must be paid.
- Frank Zappa